I’m working with this recently. It was use to interpolate the unmeasured data in environment. I asked chatgpt to explain the algorithm for me.
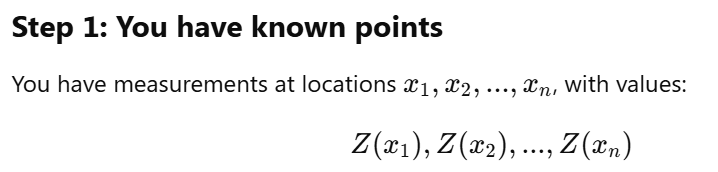
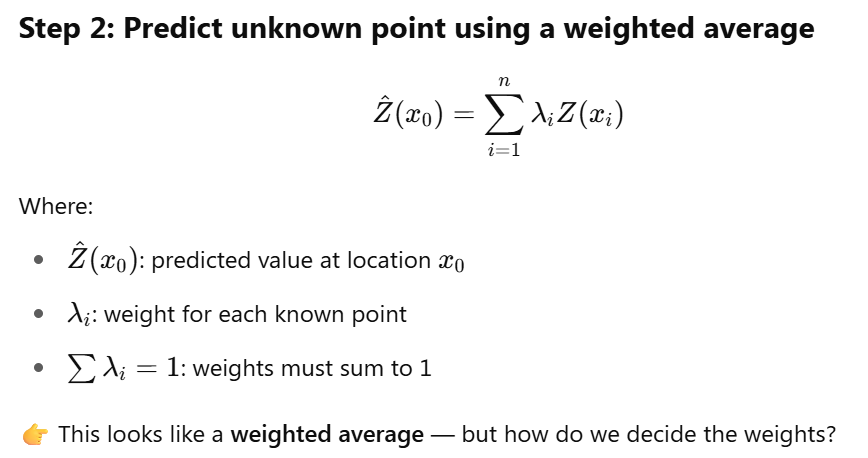
How are the weights chosen?
Kriging looks at
– Distance: closer points are more relevant
– Spatial correlation: how similar nearby points tend to be (modeled by a variogram)
The variogram describes how values “change” with distance. For example:
If points 1 meter apart are almost always similar → strong correlation
If points quickly become unrelated as you move away → weak correlation
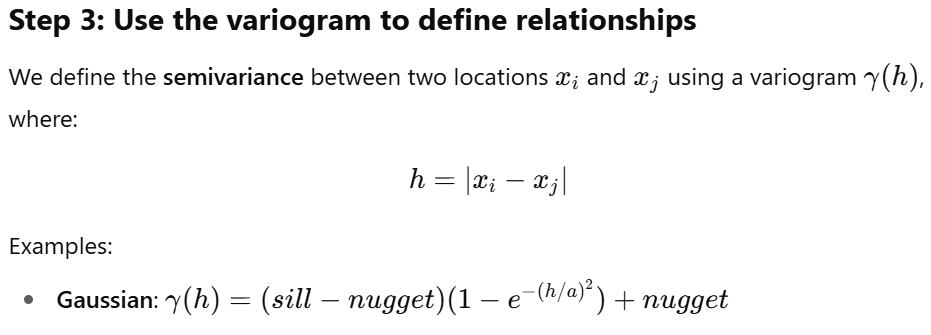
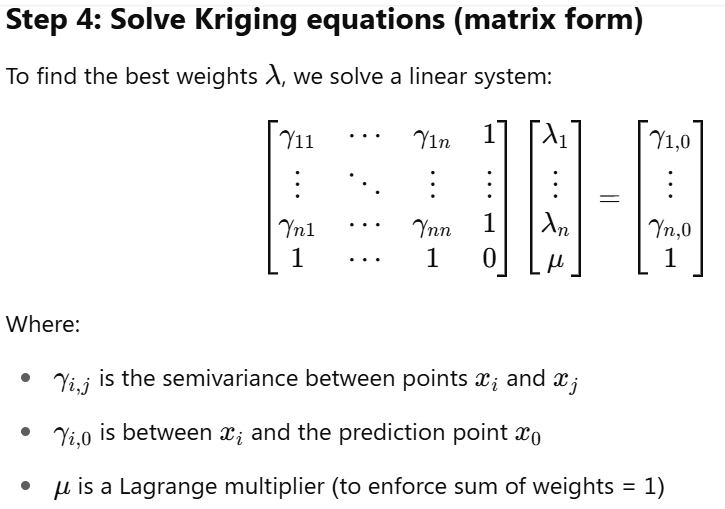
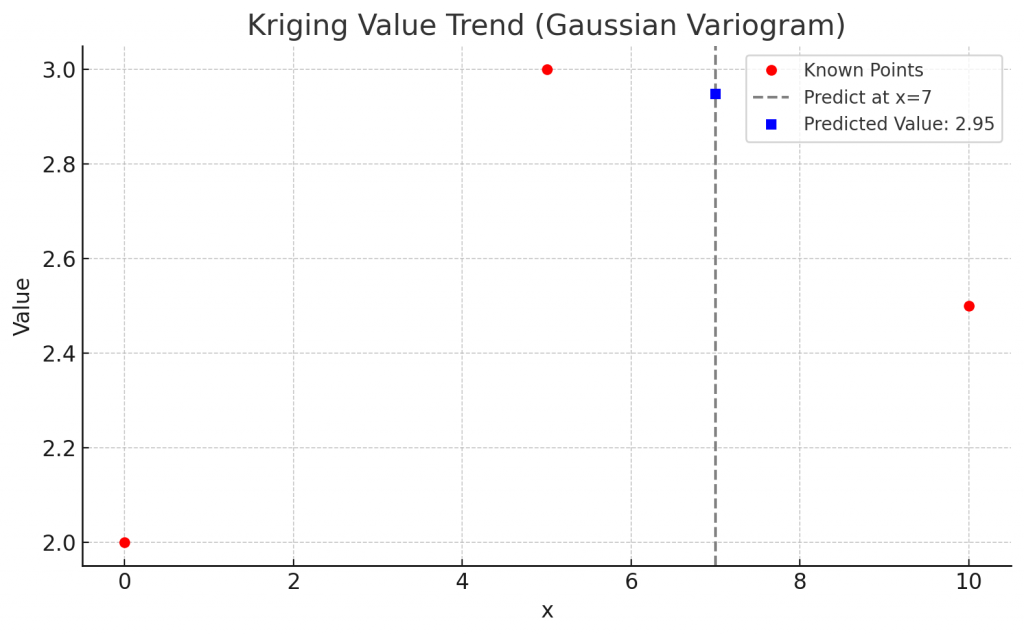
from pykrige.ok import OrdinaryKriging
OK = OrdinaryKriging(
x, y, values,
variogram_model='gaussian',
variogram_parameters=None,
nlags=6,
weight=True,
enable_plotting=False,
coordinates_type='euclidean'
)
z, ss = OK.execute('grid', gridx, gridy)
z[z < 0] = np.nan # post-process results by masking negatives
variogram_model: controls how spatial correlation decays including linear, power, gaussian(default), spherical, exponential
variogram_parameters = {‘sill’: 0.8, ‘range’: 10, ‘nugget’: 0.1}
nlags: controls how many lag bins are used to calculate the experimental variogram. More bins is more smoother fitting, but slower.
If weight is True
, It weight the variogram fitting by number of point pairs per lag.
coordinates_type
-euclidean: x, y in linear units (e.g., meters)
-geographic: lat/lon in degrees
Execute option: grid
for mesh, points
for specific locations
#If you want even more control (e.g., kernels, noise, trend), use GaussianProcessRegressor:
from sklearn.gaussian_process import GaussianProcessRegressor
from sklearn.gaussian_process.kernels import RBF, WhiteKernel, ConstantKernel
kernel = ConstantKernel() * RBF(length_scale=10.0) + WhiteKernel(noise_level=0.1)
gpr = GaussianProcessRegressor(kernel=kernel)